Data Analysis and the Use of Artificial Intelligence in Enhancing E-commerce
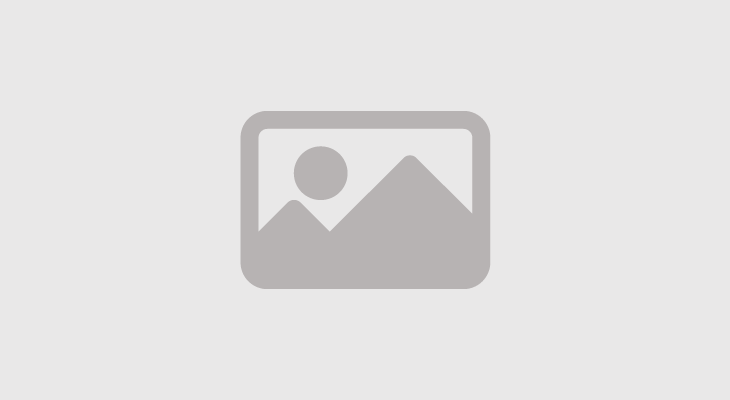
"Data analysis and the use of artificial intelligence are two crucial elements in the development and enhancement of e-commerce. Data analysis helps us better understand consumer behavior and needs, while artificial intelligence allows us to improve the shopping experience and increase operational efficiency. In this context, we will explore how these two elements can be leveraged to achieve success in the world of e-commerce."
"The Role of Data Analysis in Improving E-commerce:
Data analysis plays a vital role in enhancing and developing e-commerce. This role can be detailed as follows:
1. Understanding Customers and Their Behavior:
Data analysis can provide valuable insights into consumer behavior. By tracking customer purchasing patterns, analyzing their interaction with the website, and understanding their preferences and dislikes, e-commerce businesses can formulate better strategies to meet customer needs, increase conversion rates, and foster loyalty.
2. Enhancing User Experience:
With data analysis, the shopping experience can be personalized for each individual customer. This enables the delivery of tailored products and offers that precisely match the customer's interests and needs, increasing the likelihood of completing a transaction.
3. Inventory Management Improvement:
Data analysis helps e-commerce businesses gain a better understanding of prevalent demand patterns for products. This allows them to optimize inventory management and plan inventory more accurately, thereby avoiding sales losses due to stock shortages or surpluses.
4. Enhancing Marketing Strategies:
Through data analysis, e-commerce businesses can closely monitor the performance of marketing campaigns. This enables the identification of the most effective advertisements and platforms and better allocation of the budget towards campaigns that yield the highest return on investment.
5. Predicting Future Needs:
Data analysis can predict future customer needs and market trends, aiding e-commerce businesses in planning for a better future. It helps them provide the right products and services at the right time.
6. Fraud Prevention and Security Assurance:
Data analysis also enhances the security of e-commerce by detecting fraudulent and suspicious activities based on behavioral patterns and personal data. In summary, data analysis significantly contributes to improving e-commerce by enabling evidence-based decision-making and enhancing customer interactions, thus increasing efficiency and profitability in this increasingly competitive digital sector.
How Does Artificial Intelligence Enhance the Online Shopping Experience?
Artificial intelligence (AI) plays a prominent role in enhancing the online shopping experience by providing significant advantages and improvements. Here's how it accomplishes this in detail:
1. Product Recommendations:
AI utilizes machine learning algorithms to understand customer preferences and interests. Based on this information, AI can make personalized product recommendations for each customer. This streamlines the process of finding relevant products, making it quicker and more effective.
2. Personalized Interaction:
AI can offer a personalized interaction experience for customers. For instance, it can respond to customer inquiries, assist in product selection, and provide advice on the best deals and offers.
3. Rapid Data Analysis:
AI can process large volumes of data more quickly and accurately than humans. This allows for effective analysis of customer details and behavior, leading to improved services and recommendations.
4. Voice and Image Utilization:
Advancements in deep learning techniques enable AI to enable customers to search for products using voice commands or even images. AI can analyze images and understand spoken words, making online shopping more convenient and seamless.
5. Predictive Insights:
AI can predict future customer behavior and needs, enabling businesses to provide products and offers at the right time.
6. Fraud Detection:
AI can identify fraudulent activities based on customer behavior and transaction patterns, contributing to transaction security and fraud prevention.
7. Continuous Improvement:
AI can analyze customer interactions and responses, using this information to continuously enhance offers and services. This aids in improving customer satisfaction and loyalty. In these ways, artificial intelligence plays a crucial role in enhancing the online shopping experience by making it more personalized, convenient, and efficient, benefiting both customers and retailers alike."
"Using Data and Machine Learning to Improve Shipping and Inventory Management:
It is an essential part of successful e-commerce strategies. This utilization contributes to enhancing the efficiency and accuracy of inventory management and shipping processes, providing a better customer experience, and increasing company profitability. Here's a detailed breakdown of how this is achieved:
1. Anticipating Needs:
- Machine learning is used to analyze historical and behavioral customer data, as well as past purchasing patterns. - Based on this data, the system can predict which products customers will demand in the future. - This helps in stocking common products in larger quantities and quickly delivering them when needed.
2. Enhancing Inventory Distribution:
- Data is used to assess the historical performance of inventory and identify products that sell faster and generate higher profits. - Inventory can be better allocated to strike a balance between inventory levels and storage costs.
3. Improving Shipping Operations:
- Machine learning can optimize the planning and scheduling of shipping operations based on expected demand and delivery locations. - It can improve the selection of transportation methods and the distribution of shipments more efficiently.
4. Cost Savings in Shipping:
- Data analysis is used to examine shipping costs and available options for the company. - More economical ways of shipping products can be found, reducing shipping costs.
5. Predicting Halts and Issues:
- Data and machine learning can predict potential problems in shipping and inventory operations. - Early corrective actions can be taken to avoid halts and ensure operational continuity.
6. Enhancing Customer Experience:
- By adjusting supply and expediting shipping, machine learning can improve the customer experience by delivering products promptly. - Avoiding delays and providing reliable delivery service increases customer satisfaction and loyalty. By using data and machine learning to enhance shipping and inventory management, companies can achieve significant improvements in operational efficiency and operating costs, thus increasing their competitiveness and delivering better services to their customers.
Smart Data-Driven Strategies for Digital Marketing Targeting:
Smart data-driven strategies play a crucial role in achieving success in the digital age. They help companies maximize the value of data to understand their audience and effectively guide marketing efforts. Here's a detailed breakdown of these strategies:
1. Customer-Centric Approach:
- Marketing strategies should be customer-centric. Use data to precisely understand customer needs and preferences. - Create customer personas to define the characteristics of your target audience more accurately.
2. Comprehensive Data Collection and Analysis:
- Gather data from multiple sources, such as websites, social media, email, and e-commerce. - Use data analysis tools to understand behavior, trends, and interactions with content.
3. Customized Offers and Content:
- Utilize data to create personalized content and marketing offers for each individual or persona. - Target offers based on individual interests and preferences.
4. Automation in Marketing:
- Use marketing automation systems to collect and analyze data and prepare automatic responses. - These systems enable sending targeted messages at the right time.
5. Leverage Machine Learning and Artificial Intelligence:
- Apply machine learning and artificial intelligence techniques to analyze data quickly and with high precision. - Benefit from predictive models to guide marketing based on customer behavior expectations.
6. Continuous Performance Evaluation and Improvement:
- Continuously measure marketing campaign performance and analyze results. - Use this data to fine-tune and enhance your strategy for improved efficiency.
7. Maintain Privacy and Security:
- Adhere to best practices for data privacy and security when collecting and using customer data. - Comply with relevant local and international regulations.
8. Develop Multichannel Strategies:
- Direct marketing efforts across various digital channels, such as social media, email, and web content. - Adopt integrated strategies to effectively reach your audience. By employing these smart strategies, companies can achieve significant success in guiding digital marketing to precisely meet the needs and preferences of their customers, increasing the effectiveness of their marketing campaigns in the digital marketplace."
"Data Analysis and Artificial Intelligence in Fraud Prevention and Transaction Security:
Data analysis and artificial intelligence play a vital role in combating fraud and enhancing transaction security online. These technologies are an integral part of the security and protection strategies followed by companies and organizations. Here's how it's done in detail:
1. Fraud Detection:
- Data analysis enables monitoring of unusual or suspicious behavioral patterns. - Machine learning techniques are used to analyze data and detect any suspicious activities, such as unusual payment transactions or unknown activities.
2. Risk Classification:
- Data analysis and artificial intelligence help in classifying risks and identifying high-risk operations. - Predictive models can be created to assess the potential risk level of transactions.
3. Detecting Fraudulent Patterns:
- Artificial intelligence can analyze data to discover possible fraudulent patterns. - For example, it can analyze patterns of credit card usage and detect any unusual activity, such as multiple purchases from different websites simultaneously.
4. Improving Identity Verification:
- Machine learning can be used to enhance identity verification processes. - This includes the use of facial recognition, biometrics, and speech recognition to verify users' identities more effectively and securely.
5. Enhancing Timeliness:
- Artificial intelligence can analyze data instantly and provide immediate warnings of suspicious activities. - This helps in mitigating the negative effects of fraudulent activities before they occur.
6. Machine Learning for Adapting to Threats:
- Machine learning can continuously improve security models based on new fraud patterns and threats. - It helps in enhancing security defenses sustainably.
7. Financial Data Analysis:
- Data analysis can assist companies in analyzing their financial transactions and detecting potential fraud in financial operations.
8. Compliance with Regulations:
- Data analysis and artificial intelligence can be used to ensure compliance with financial regulations and relevant laws. These smart strategies combine data analysis and artificial intelligence to provide better security for digital transactions and effectively combat fraud. They help protect customers and businesses from cyber threats and enhance consumer trust in e-commerce and digital payments.
Predictive Data Analysis and Consumer Behavior Forecasting:
This is an important field in data science and data analysis. It aims to understand and predict how individuals and customers will behave in the future based on historical data and available information. Here's how it's achieved in detail:
1. Data Collection:
- Predictive data analysis starts with collecting relevant data. This data may include historical customer records, past transactions, and interactions across websites and social media.
2. Data Cleaning and Initial Analysis:
- Data must be cleaned of errors, missing values, and duplicates. - Initial analysis involves basic statistical analysis and exploring simple patterns in the data.
3. Predictive Data Analysis:
- Predictive data analysis techniques, such as predictive modeling and machine learning, are used to deeply analyze the data. - These models are directed towards predicting or forecasting potential future behavior.
4. Utilizing Predictive Models:
- Once predictive models are developed, they can be used to predict future customer behavior. - Marketing efforts, advertising design, and offers can be directed based on these predictions.
5. Evaluation and Continuous Improvement:
- Continuous evaluation of the predictive models' performance and accuracy is essential. - Models can be improved based on new data and customer responses to the actions taken.
6. Practical Applications:
- Predictions of consumer behavior can be applied to a variety of practical applications, such as improving targeted marketing, enhancing customer experiences, and optimizing inventory management.
7. Privacy and Security:
- Companies must adhere to privacy and security standards when using customer data for analysis and prediction."
"Data Analysis and Customer Personalization:
Data analysis and customer personalization are fundamental concepts in the fields of marketing and customer relationship management. This concept helps companies make the most of the available data to provide customized experiences and achieve customer satisfaction. Here's how it's done in detail:
1. Data Collection and Cleaning:
- The process starts with collecting data from various sources such as websites, e-commerce, social media, phone calls, and emails. - Data must be cleaned and formatted to ensure accuracy and effective utilization.
2. Data Analysis:
- Data analysis involves using various data analysis techniques such as machine learning, behavioral analysis, clustering, and classification. - The analysis aims to extract patterns and insights from data that reveal customer preferences and needs.
3. Customer Segmentation:
- Customers are divided into different categories based on common information such as age, gender, geographic location, and shopping behavior. - This helps in better understanding who the customers are and what they desire.
4. Targeting Offers and Services:
- Based on data analysis and customer segmentation, offers and services can be personalized for each customer category. - This includes sending targeted marketing messages and special offers to each group.
5. Continuous Interaction:
- Tracking customer responses and interactions with offers and services is essential. - This data can be analyzed to adjust and improve offers based on customer behavior.
6. Swift Response:
- When customers interact with offers or provide feedback, quick responses should be provided, and this information should be used to better meet their needs.
7. Performance Measurement and Continuous Improvement:
- The performance of the personalized offers strategy should be measured, and its success monitored.
Challenges and Future Trends in Data Analysis and Artificial Intelligence in E-commerce:
Data analysis and artificial intelligence have transformed and rapidly evolved e-commerce. However, there are challenges and future trends that significantly impact this field. I will explain these challenges and trends in detail:
Challenges:
1. Data Protection and Privacy:
- With the advancement of data analysis and the use of personal data, concerns about data protection and privacy are increasing. - Tightening regulations and laws (such as GDPR in Europe) place additional pressure on companies to ensure data security and respect privacy.
2. Resource and Skill Shortage:
- Many companies face a shortage of human resources and specialized skills in data analysis and artificial intelligence. - Training and hiring the necessary skills pose a challenge with the increasing demand for specialists.
3. Data Complexity:
- E-commerce data is becoming more complex with its increasing volume and diversity. - Analyzing and cleaning this data requires significant effort and advanced techniques.
4. Technical Challenges:
- Modern data analysis and artificial intelligence techniques require high computational capabilities. - Technical challenges include infrastructure development and continuous improvement in performance and security.
Future Trends:
1. Machine Learning and Artificial Intelligence:
- Increased use of machine learning and artificial intelligence is expected in personalized marketing and targeting. - Predictive models, robots, and artificial intelligence can enhance customer experiences and increase management efficiency.
2. Detailed and Behavioral Analysis:
- Analytics will be developed to understand deeper details about customer behavior and preferences. - Behavioral analysis can lead to more accurate targeting and more personalized experiences.
3. Social Analysis and Engagement:
- Increased use of social media for behavioral analysis and customer response is foreseen.
summary..
In conclusion, data analysis and the use of artificial intelligence represent crucial factors in enhancing e-commerce, making it more efficient, and improving the customer experience. This integration of technology and data helps gain a better understanding of customer needs and enables more precise and personalized targeting of offers and services. With the continuous evolution of data analysis and artificial intelligence, we will witness greater improvements in the field of e-commerce, including better customer expectations, increased operational efficiency, and more business successes. Companies must consistently leverage these technologies to enhance their offerings and stay competitive in the competitive world of e-commerce.
Comment / Reply From
You May Also Like
منشورات شائعة
Newsletter
Subscribe to our mailing list to get the new updates!